Creating the Virtual Human Twin across scales
Virtual Human Twins that capture multiple scales offer unique possibilities to replace many animal experiments according to the 3R Principles. Digital twins of animal and other experiments can further contribute to reducing and refining animal experiments. At the Virtual Human Twin Core Unit, we develop computational models of small units such as cell distribution and cell fate in biopsies to microenvironments in tumors and organoids or even larger entities such as whole organs such as the heart. AI technologies can aid in personalizing and accelerating simulations with these computational models but also AI approaches can be enabled through large scale simulation studies in turn. Our expertise comprises technologies from computational image analysis, 3D tissue modeling, biomechanics and bioelectricity modeling and simulation, molecular biodiversity to biomedical data science.
A Virtual Human Twin is a personalized computational model of an individual patient. More general, a digital twin model mimics the structure and behavior of an individual or a technical object (e.g. a power plant) according to the definition of the AIAA Digital Engineering Integration Committee. Sometimes digital twin models are built for decision support in one critical timestep. While this is currently the most wide-spread use case in the medical field, digital twins in general are dynamically updated from the physical twin throughout their life-cycle, a vision for future developments also in the HealthTech field.
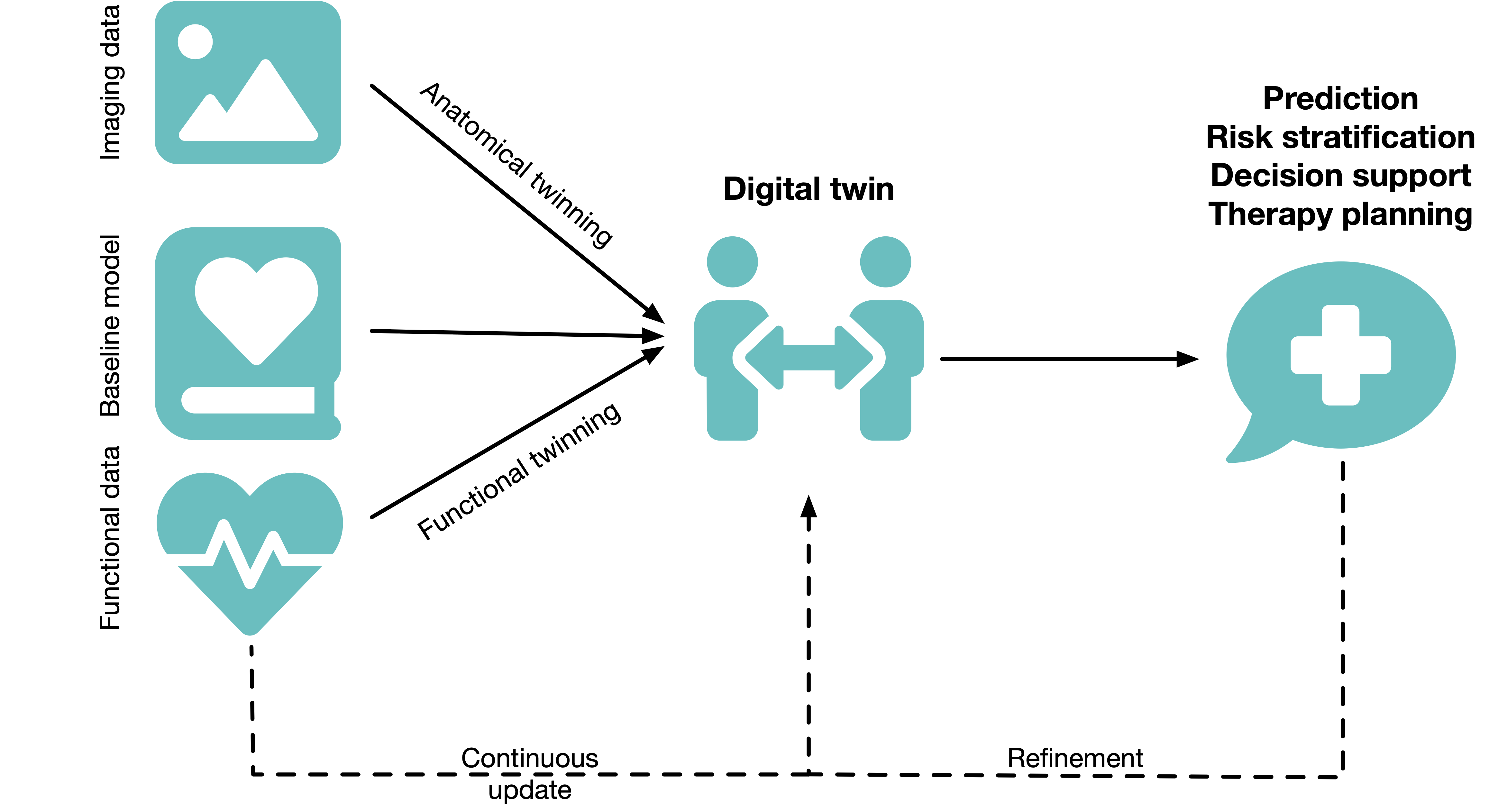
Virtual Human Twins are used for individual risk prediction, decision support, and therapy planning. For example, different options for interventions can be evaluated in silico before deciding for the approach to be applied to the patient following the personalized medicine vision.
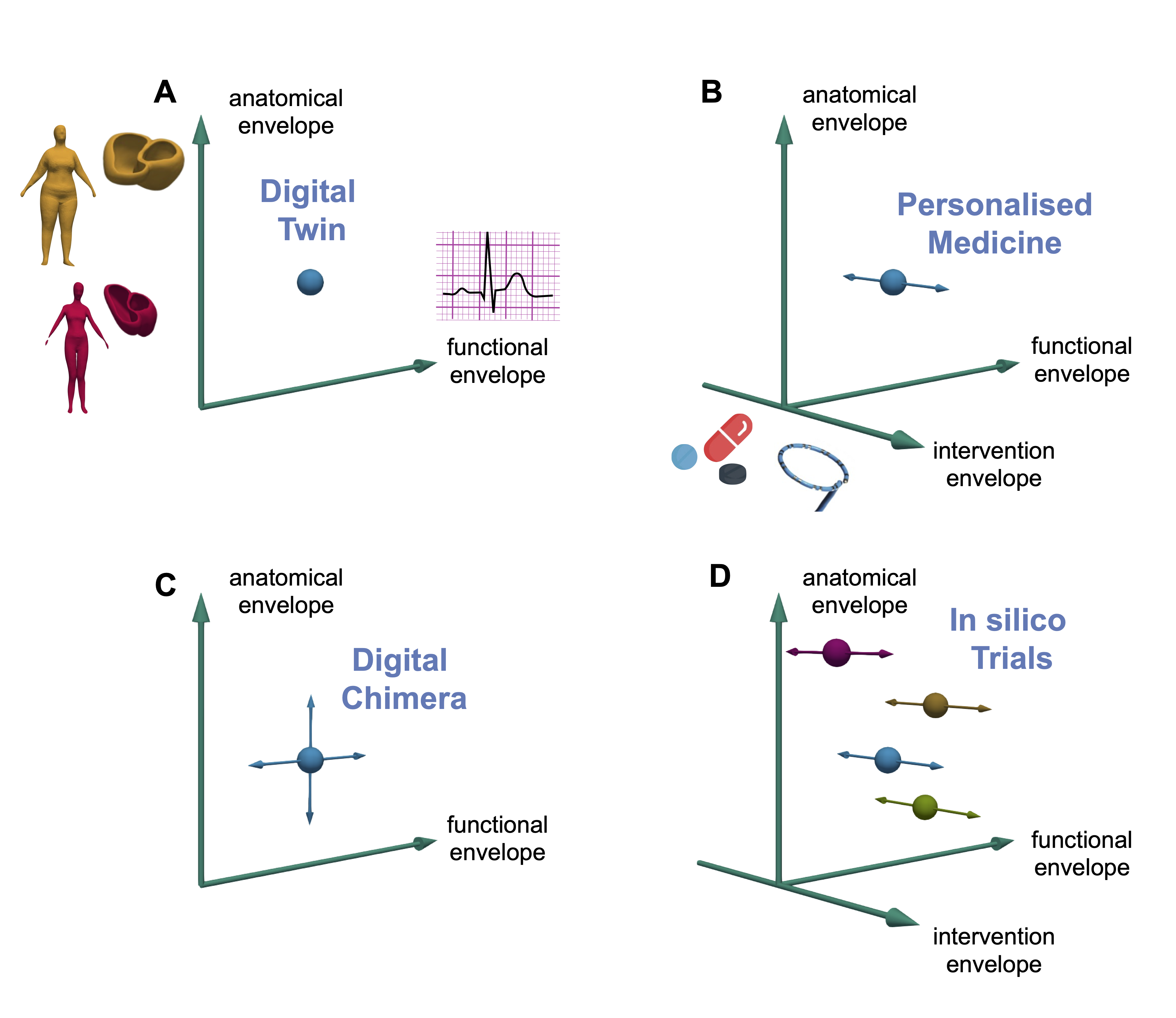
One field in which Virtual Human Twins are quite advanced already is cardiology. We outline current applications and future visions in a chapter of the book Innovative Treatment Strategies for Clinical Electrophysiology. To exploit modeling & simulation technologies, we need to formulate the biological function in terms of mathematical equations as presented below for the example of cardiac electrophysiology across the cellular, tissue, organ and body scales.
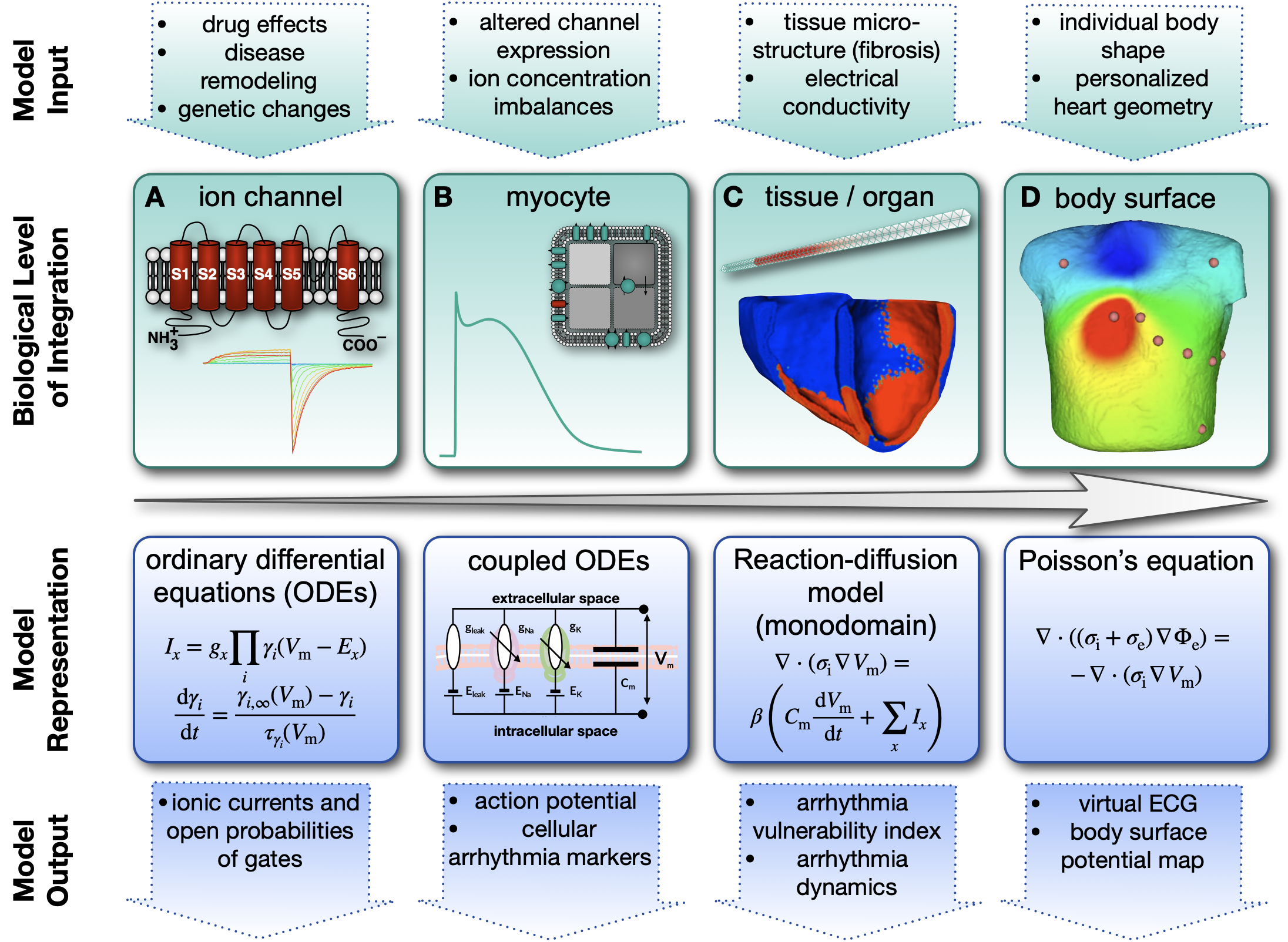
integrated cell models (B) and tissue level models (C) to the body surface and electrocardiogram (D). The simulation system allows investigating what-if scenarios by changing input parameters of the model (top row) and analysing the effect on simulation outputs on numerous scales (bottom row).